
You could assign a number or score to each category, say 1 for furry, 2 for nude and 3 for wrinkled. If you wish to have intermediate categories, you must provide them before you make your measurements. If you also record whether they are 'furry', 'nude' or 'wrinkled', this is another nominal variable. Or you may record the number of mice that are white, brown, or brown and white.
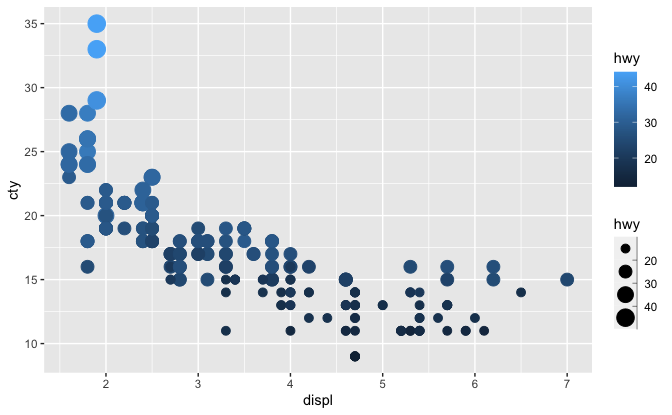
For example, fungal cultures could be classified as 'white', 'yellow' or 'green'. Thus colour is measured on the nominal scale (or categorical scale). The most important are the scale of measurement used, whether the distribution of the variable is discrete or continuous, and whether it is a primary or derived variable.Ī nominal variable (also known as a categorical variable) has a number of categories to which an observation can be assigned. There are several criteria we can use to classify variables. Or we could measure the duration, rainfall and windspeed of a storm, or the temperature, humidity and wind direction preceding it. The length is one variable, the width is another. For example, we may measure the length and width of a tooth. There can be any number of variables pertaining to an individual item or event. We can formally define a variable as a characteristic of an evaluation unit that can assume a number of different values. Thus, each type of observation we make is called a variable. If they never varied, there would be little reason to make the observations. Inevitably, we are interested in how our observations vary. The difference is the extent to which you can infer that one causes the other. Both may be measured, are subject to error, and can apply to the same item - such as a mouse. You can make observations on different aspects of an event (or a mouse), such as how big it is, when it begins (or is born, or conceived), how long it survives for, and how it affects things around it.įor their conclusions to be of practical use, scientists need to distinguish treatments they apply from results they observe. An event is something that happens, such as a storm. If we weigh it again, or weigh another mouse, that is a second observation.ĭo not confuse observations with events. The weight of a mouse is a single observation.

The evaluation unit is commonly a single individual. These are classifications or measurements made on the smallest sampling unit, known as the evaluation unit. In practice, we are interested in a number of items of information for which we use the plural of datum - data.ĭata exist as observations. But before we can proceed, we need to define a few key terms.Ī datum is a single item of information. The first obstacle to be overcome in this matter lies in describing what sort of information you are dealing with. Since the properties of their information have major implications for the validity of their subsequent analysis, this is rather a shame. Even when scientists can be persuaded to look at their (raw, unanalyzed) results, they seldom consider the properties inherent to that form of information - or how those properties might affect any statistics they may use to summarize their results.
